Review on solving the inverse problem in EEG source analysis
- PMID: 18990257
- PMCID: PMC2605581
- DOI: 10.1186/1743-0003-5-25
Review on solving the inverse problem in EEG source analysis
Abstract
In this primer, we give a review of the inverse problem for EEG source localization. This is intended for the researchers new in the field to get insight in the state-of-the-art techniques used to find approximate solutions of the brain sources giving rise to a scalp potential recording. Furthermore, a review of the performance results of the different techniques is provided to compare these different inverse solutions. The authors also include the results of a Monte-Carlo analysis which they performed to compare four non parametric algorithms and hence contribute to what is presently recorded in the literature. An extensive list of references to the work of other researchers is also provided. This paper starts off with a mathematical description of the inverse problem and proceeds to discuss the two main categories of methods which were developed to solve the EEG inverse problem, mainly the non parametric and parametric methods. The main difference between the two is to whether a fixed number of dipoles is assumed a priori or not. Various techniques falling within these categories are described including minimum norm estimates and their generalizations, LORETA, sLORETA, VARETA, S-MAP, ST-MAP, Backus-Gilbert, LAURA, Shrinking LORETA FOCUSS (SLF), SSLOFO and ALF for non parametric methods and beamforming techniques, BESA, subspace techniques such as MUSIC and methods derived from it, FINES, simulated annealing and computational intelligence algorithms for parametric methods. From a review of the performance of these techniques as documented in the literature, one could conclude that in most cases the LORETA solution gives satisfactory results. In situations involving clusters of dipoles, higher resolution algorithms such as MUSIC or FINES are however preferred. Imposing reliable biophysical and psychological constraints, as done by LAURA has given superior results. The Monte-Carlo analysis performed, comparing WMN, LORETA, sLORETA and SLF, for different noise levels and different simulated source depths has shown that for single source localization, regularized sLORETA gives the best solution in terms of both localization error and ghost sources. Furthermore the computationally intensive solution given by SLF was not found to give any additional benefits under such simulated conditions.
Figures
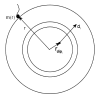
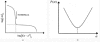
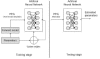
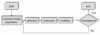
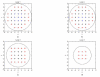
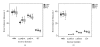
Similar articles
-
Linear distributed inverse solutions for interictal EEG source localisation.Clin Neurophysiol. 2022 Jan;133:58-67. doi: 10.1016/j.clinph.2021.10.008. Epub 2021 Nov 8. Clin Neurophysiol. 2022. PMID: 34801964
-
A recursive algorithm for the three-dimensional imaging of brain electric activity: Shrinking LORETA-FOCUSS.IEEE Trans Biomed Eng. 2004 Oct;51(10):1794-802. doi: 10.1109/TBME.2004.831537. IEEE Trans Biomed Eng. 2004. PMID: 15490826
-
Standardized shrinking LORETA-FOCUSS (SSLOFO): a new algorithm for spatio-temporal EEG source reconstruction.IEEE Trans Biomed Eng. 2005 Oct;52(10):1681-91. doi: 10.1109/TBME.2005.855720. IEEE Trans Biomed Eng. 2005. PMID: 16235654
-
Review on solving the forward problem in EEG source analysis.J Neuroeng Rehabil. 2007 Nov 30;4:46. doi: 10.1186/1743-0003-4-46. J Neuroeng Rehabil. 2007. PMID: 18053144 Free PMC article. Review.
-
Trends in EEG source localization.Electroencephalogr Clin Neurophysiol. 1998 Feb;106(2):127-37. doi: 10.1016/s0013-4694(97)00115-6. Electroencephalogr Clin Neurophysiol. 1998. PMID: 9741773 Review.
Cited by
-
The Pre-Interictal Network State in Idiopathic Generalized Epilepsies.Brain Sci. 2023 Dec 2;13(12):1671. doi: 10.3390/brainsci13121671. Brain Sci. 2023. PMID: 38137119 Free PMC article.
-
Assessing cortical network properties using TMS-EEG.Hum Brain Mapp. 2013 Jul;34(7):1652-69. doi: 10.1002/hbm.22016. Epub 2012 Feb 29. Hum Brain Mapp. 2013. PMID: 22378543 Free PMC article.
-
Methods for simultaneous EEG-fMRI: an introductory review.J Neurosci. 2012 May 2;32(18):6053-60. doi: 10.1523/JNEUROSCI.0447-12.2012. J Neurosci. 2012. PMID: 22553012 Free PMC article.
-
EEG signatures accompanying auditory figure-ground segregation.Neuroimage. 2016 Nov 1;141:108-119. doi: 10.1016/j.neuroimage.2016.07.028. Epub 2016 Jul 12. Neuroimage. 2016. PMID: 27421185 Free PMC article.
-
The impact of acute psychosocial stress on magnetoencephalographic correlates of emotional attention and exogenous visual attention.PLoS One. 2012;7(6):e35767. doi: 10.1371/journal.pone.0035767. Epub 2012 Jun 11. PLoS One. 2012. PMID: 22701552 Free PMC article.
References
-
- Whittingstall K, Stroink G, Gates L, Connolly JF, Finley A. Effects of dipole position, orientation and noise on the accuracy of EEG source localization. Biomedical Engineering Online. 2003;2 http://www.biomedical-engineering-online.com/content/2/1/14 - PMC - PubMed
-
- Pascual-Marqui RD. Review of Methods for Solving the EEG Inverse Problem. International Journal of Bioelectromagnetism. 1999;1:75–86. [Author's version]
Publication types
MeSH terms
LinkOut - more resources
Full Text Sources
Other Literature Sources