A Review of Artificial Intelligence Applications in Hematology Management: Current Practices and Future Prospects
- PMID: 35819826
- PMCID: PMC9328784
- DOI: 10.2196/36490
A Review of Artificial Intelligence Applications in Hematology Management: Current Practices and Future Prospects
Abstract
Background: Machine learning (ML) and deep learning (DL) methods have recently garnered a great deal of attention in the field of cancer research by making a noticeable contribution to the growth of predictive medicine and modern oncological practices. Considerable focus has been particularly directed toward hematologic malignancies because of the complexity in detecting early symptoms. Many patients with blood cancer do not get properly diagnosed until their cancer has reached an advanced stage with limited treatment prospects. Hence, the state-of-the-art revolves around the latest artificial intelligence (AI) applications in hematology management.
Objective: This comprehensive review provides an in-depth analysis of the current AI practices in the field of hematology. Our objective is to explore the ML and DL applications in blood cancer research, with a special focus on the type of hematologic malignancies and the patient's cancer stage to determine future research directions in blood cancer.
Methods: We searched a set of recognized databases (Scopus, Springer, and Web of Science) using a selected number of keywords. We included studies written in English and published between 2015 and 2021. For each study, we identified the ML and DL techniques used and highlighted the performance of each model.
Results: Using the aforementioned inclusion criteria, the search resulted in 567 papers, of which 144 were selected for review.
Conclusions: The current literature suggests that the application of AI in the field of hematology has generated impressive results in the screening, diagnosis, and treatment stages. Nevertheless, optimizing the patient's pathway to treatment requires a prior prediction of the malignancy based on the patient's symptoms or blood records, which is an area that has still not been properly investigated.
Keywords: artificial intelligence; cancer; deep learning; hematology; machine learning; malignancy; management; oncology; prediction.
©Yousra El Alaoui, Adel Elomri, Marwa Qaraqe, Regina Padmanabhan, Ruba Yasin Taha, Halima El Omri, Abdelfatteh EL Omri, Omar Aboumarzouk. Originally published in the Journal of Medical Internet Research (https://www.jmir.org), 12.07.2022.
Conflict of interest statement
Conflicts of Interest: None declared.
Figures
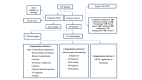
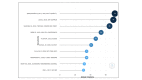
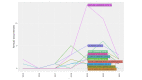
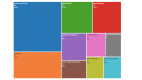
Similar articles
-
Artificial Intelligence in Hematology: Current Challenges and Opportunities.Curr Hematol Malig Rep. 2020 Jun;15(3):203-210. doi: 10.1007/s11899-020-00575-4. Curr Hematol Malig Rep. 2020. PMID: 32239350 Review.
-
Artificial intelligence in spine care: current applications and future utility.Eur Spine J. 2022 Aug;31(8):2057-2081. doi: 10.1007/s00586-022-07176-0. Epub 2022 Mar 27. Eur Spine J. 2022. PMID: 35347425 Review.
-
Data-driven modeling and prediction of blood glucose dynamics: Machine learning applications in type 1 diabetes.Artif Intell Med. 2019 Jul;98:109-134. doi: 10.1016/j.artmed.2019.07.007. Epub 2019 Jul 26. Artif Intell Med. 2019. PMID: 31383477 Review.
-
Clinical Applications of Artificial Intelligence and Machine Learning in Children with Cleft Lip and Palate-A Systematic Review.Int J Environ Res Public Health. 2022 Aug 31;19(17):10860. doi: 10.3390/ijerph191710860. Int J Environ Res Public Health. 2022. PMID: 36078576 Free PMC article. Review.
-
Applications of artificial intelligence in urologic oncology.Investig Clin Urol. 2024 May;65(3):202-216. doi: 10.4111/icu.20230435. Investig Clin Urol. 2024. PMID: 38714511 Free PMC article. Review.
Cited by
-
Applications of artificial intelligence-assisted retinal imaging in systemic diseases: A literature review.Saudi J Ophthalmol. 2023 Oct 19;37(3):185-192. doi: 10.4103/sjopt.sjopt_153_23. eCollection 2023 Jul-Sep. Saudi J Ophthalmol. 2023. PMID: 38074306 Free PMC article.
-
Surveying Hematologists' Perceptions and Readiness to Embrace Artificial Intelligence in Diagnosis and Treatment Decision-Making.Cureus. 2023 Nov 26;15(11):e49462. doi: 10.7759/cureus.49462. eCollection 2023 Nov. Cureus. 2023. PMID: 38152821 Free PMC article.
-
CDC-NET: a cell detection and confirmation network of bone marrow aspirate images for the aided diagnosis of AML.Med Biol Eng Comput. 2024 Feb;62(2):575-589. doi: 10.1007/s11517-023-02955-3. Epub 2023 Nov 12. Med Biol Eng Comput. 2024. PMID: 37953336
-
Promising Therapeutic Strategies for Hematologic Malignancies: Innovations and Potential.Molecules. 2024 Sep 9;29(17):4280. doi: 10.3390/molecules29174280. Molecules. 2024. PMID: 39275127 Free PMC article. Review.
-
Integrating AI and ML in Myelodysplastic Syndrome Diagnosis: State-of-the-Art and Future Prospects.Cancers (Basel). 2023 Dec 22;16(1):65. doi: 10.3390/cancers16010065. Cancers (Basel). 2023. PMID: 38201493 Free PMC article. Review.
References
-
- Das M, Kumar Saha P. A Comprehensive Study of Machine Learning algorithms for Predicting Leukemia Based on Biomedical Data. International Conference on Innovation in Engineering and Technology; December 23-24, 2019; Dhaka, Bangladesh. 2020. Dec 21, pp. 1–5. - DOI
-
- Acharya V, Ravi V, Pham TD, Chakraborty C. Peripheral Blood Smear Analysis Using Automated Computer-Aided Diagnosis System to Identify Acute Myeloid Leukemia. IEEE Trans. Eng. Manage. 2021:1–14. doi: 10.1109/tem.2021.3103549. - DOI
-
- Sipes R, Li D. Using Convolutional Neural Networks for Automated Fine Grained Image Classification of Acute Lymphoblastic Leukemia. 2018 3rd International Conference on Computational Intelligence and Applications (ICCIA); July 28-30, 2018; Hong Kong, China. 2019. May, pp. 157–161. - DOI
-
- Ratley A, Minj J, Patre P. Leukemia Disease Detection and Classification Using Machine Learning Approaches: A Review. 2020 First International Conference on Power, Control and Computing Technologies (ICPC2T); January 3-5, 2020; Raipur, Chhattisgarh, India. 2020. pp. 161–165. - DOI
Publication types
MeSH terms
LinkOut - more resources
Full Text Sources