Evaluation of different biological data and computational classification methods for use in protein interaction prediction
- PMID: 16450363
- PMCID: PMC3250929
- DOI: 10.1002/prot.20865
Evaluation of different biological data and computational classification methods for use in protein interaction prediction
Abstract
Protein-protein interactions play a key role in many biological systems. High-throughput methods can directly detect the set of interacting proteins in yeast, but the results are often incomplete and exhibit high false-positive and false-negative rates. Recently, many different research groups independently suggested using supervised learning methods to integrate direct and indirect biological data sources for the protein interaction prediction task. However, the data sources, approaches, and implementations varied. Furthermore, the protein interaction prediction task itself can be subdivided into prediction of (1) physical interaction, (2) co-complex relationship, and (3) pathway co-membership. To investigate systematically the utility of different data sources and the way the data is encoded as features for predicting each of these types of protein interactions, we assembled a large set of biological features and varied their encoding for use in each of the three prediction tasks. Six different classifiers were used to assess the accuracy in predicting interactions, Random Forest (RF), RF similarity-based k-Nearest-Neighbor, Naïve Bayes, Decision Tree, Logistic Regression, and Support Vector Machine. For all classifiers, the three prediction tasks had different success rates, and co-complex prediction appears to be an easier task than the other two. Independently of prediction task, however, the RF classifier consistently ranked as one of the top two classifiers for all combinations of feature sets. Therefore, we used this classifier to study the importance of different biological datasets. First, we used the splitting function of the RF tree structure, the Gini index, to estimate feature importance. Second, we determined classification accuracy when only the top-ranking features were used as an input in the classifier. We find that the importance of different features depends on the specific prediction task and the way they are encoded. Strikingly, gene expression is consistently the most important feature for all three prediction tasks, while the protein interactions identified using the yeast-2-hybrid system were not among the top-ranking features under any condition.
(c) 2006 Wiley-Liss, Inc.
Figures
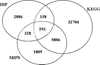
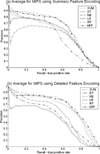
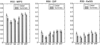
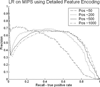
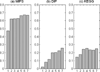
Similar articles
-
Probabilistic prediction and ranking of human protein-protein interactions.BMC Bioinformatics. 2007 Jul 5;8:239. doi: 10.1186/1471-2105-8-239. BMC Bioinformatics. 2007. PMID: 17615067 Free PMC article.
-
A mixture of feature experts approach for protein-protein interaction prediction.BMC Bioinformatics. 2007;8 Suppl 10(Suppl 10):S6. doi: 10.1186/1471-2105-8-S10-S6. BMC Bioinformatics. 2007. PMID: 18269700 Free PMC article.
-
Effectively Identifying Compound-Protein Interactions by Learning from Positive and Unlabeled Examples.IEEE/ACM Trans Comput Biol Bioinform. 2018 Nov-Dec;15(6):1832-1843. doi: 10.1109/TCBB.2016.2570211. Epub 2016 May 18. IEEE/ACM Trans Comput Biol Bioinform. 2018. PMID: 28113437
-
Predicting membrane protein types using various decision tree classifiers based on various modes of general PseAAC for imbalanced datasets.J Theor Biol. 2017 Dec 21;435:208-217. doi: 10.1016/j.jtbi.2017.09.018. Epub 2017 Sep 20. J Theor Biol. 2017. PMID: 28941868 Review.
-
Application of Machine Learning Approaches for Protein-protein Interactions Prediction.Med Chem. 2017;13(6):506-514. doi: 10.2174/1573406413666170522150940. Med Chem. 2017. PMID: 28530547 Review.
Cited by
-
Atypical cytostatic mechanism of N-1-sulfonylcytosine derivatives determined by in vitro screening and computational analysis.Invest New Drugs. 2008 Apr;26(2):97-110. doi: 10.1007/s10637-007-9084-1. Epub 2007 Sep 27. Invest New Drugs. 2008. PMID: 17898928
-
Predicting protein targets for drug-like compounds using transcriptomics.PLoS Comput Biol. 2018 Dec 7;14(12):e1006651. doi: 10.1371/journal.pcbi.1006651. eCollection 2018 Dec. PLoS Comput Biol. 2018. PMID: 30532261 Free PMC article.
-
Exploring Machine Learning Algorithms to Unveil Genomic Regions Associated With Resistance to Southern Root-Knot Nematode in Soybeans.Front Plant Sci. 2022 May 3;13:883280. doi: 10.3389/fpls.2022.883280. eCollection 2022. Front Plant Sci. 2022. PMID: 35592556 Free PMC article.
-
Supervised learning and prediction of physical interactions between human and HIV proteins.Infect Genet Evol. 2011 Jul;11(5):917-23. doi: 10.1016/j.meegid.2011.02.022. Epub 2011 Mar 5. Infect Genet Evol. 2011. PMID: 21382517 Free PMC article.
-
Computational prediction of the human-microbial oral interactome.BMC Syst Biol. 2014 Feb 27;8:24. doi: 10.1186/1752-0509-8-24. BMC Syst Biol. 2014. PMID: 24576332 Free PMC article.
References
-
- Bader GD, Hogue CW. Analyzing yeast protein–protein interaction data obtained from different sources. Nat Biotechnol. 2003;20:991–997. - PubMed
-
- von Mering C, Krause R, Snel B, Cornell M, Oliver S, Fields S, Bork P. Comparative assessment of large-scale data sets of protein–protein interactions. Nature. 2002;417:399–403. - PubMed
-
- Uetz P, Giot L, Cagney G, Mansfield TA, Judson RS, Knight JR, Lockshon D, Narayan V, Srinivasan M, Pochart P, Qureshi-Emili A, Li Y, Godwin B, Conover D, Kalbfleisch T, Vijayadamodar G, Yang M, Johnston M, Fields S, Rothberg JM. A comprehensive analysis of protein–protein interactions in Saccharomyces cerevisiae. Nature. 2000;403:623–627. - PubMed
-
- Gavin AC, Bosche M, Krause R, Grandi P, Marzioch M, Bauer A, Schultz J, Rick JM, Michon AM, Cruciat CM, Remor M, Hofert C, Schelder M, Brajenovic M, Ruffner H, Merino A, Klein K, Hudak M, Dickson D, Rudi T, Gnau V, Bauch A, Bastuck S, Huhse B, Leutwein C, Heurtier MA, Copley RR, Edelmann A, Querfurth E, Rybin V, Drewes G, Raida M, Bouwmeester T, Bork P, Seraphin B, Kuster B, Neubauer G, Superti-Furga G. Functional organization of the yeast proteome by systematic analysis of protein complexes. Nature. 2002;415:141–147. - PubMed
Publication types
MeSH terms
Grants and funding
LinkOut - more resources
Full Text Sources
Molecular Biology Databases