Data reduction for spectral clustering to analyze high throughput flow cytometry data
- PMID: 20667133
- PMCID: PMC2923634
- DOI: 10.1186/1471-2105-11-403
Data reduction for spectral clustering to analyze high throughput flow cytometry data
Abstract
Background: Recent biological discoveries have shown that clustering large datasets is essential for better understanding biology in many areas. Spectral clustering in particular has proven to be a powerful tool amenable for many applications. However, it cannot be directly applied to large datasets due to time and memory limitations. To address this issue, we have modified spectral clustering by adding an information preserving sampling procedure and applying a post-processing stage. We call this entire algorithm SamSPECTRAL.
Results: We tested our algorithm on flow cytometry data as an example of large, multidimensional data containing potentially hundreds of thousands of data points (i.e., "events" in flow cytometry, typically corresponding to cells). Compared to two state of the art model-based flow cytometry clustering methods, SamSPECTRAL demonstrates significant advantages in proper identification of populations with non-elliptical shapes, low density populations close to dense ones, minor subpopulations of a major population and rare populations.
Conclusions: This work is the first successful attempt to apply spectral methodology on flow cytometry data. An implementation of our algorithm as an R package is freely available through BioConductor.
Figures

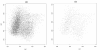
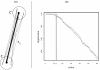
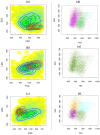
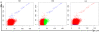
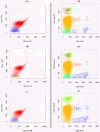
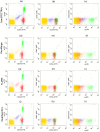
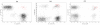
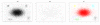
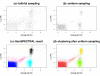
Similar articles
-
Ultrafast clustering of single-cell flow cytometry data using FlowGrid.BMC Syst Biol. 2019 Apr 5;13(Suppl 2):35. doi: 10.1186/s12918-019-0690-2. BMC Syst Biol. 2019. PMID: 30953498 Free PMC article.
-
Misty Mountain clustering: application to fast unsupervised flow cytometry gating.BMC Bioinformatics. 2010 Oct 9;11:502. doi: 10.1186/1471-2105-11-502. BMC Bioinformatics. 2010. PMID: 20932336 Free PMC article.
-
SWIFT-scalable clustering for automated identification of rare cell populations in large, high-dimensional flow cytometry datasets, part 2: biological evaluation.Cytometry A. 2014 May;85(5):422-33. doi: 10.1002/cyto.a.22445. Epub 2014 Feb 14. Cytometry A. 2014. PMID: 24532172 Free PMC article.
-
Full spectrum flow cytometry in the clinical laboratory.Int J Lab Hematol. 2023 Jun;45 Suppl 2(Suppl 2):44-49. doi: 10.1111/ijlh.14098. Epub 2023 May 21. Int J Lab Hematol. 2023. PMID: 37211417 Free PMC article. Review.
-
Imaging flow cytometry analysis of intracellular pathogens.Methods. 2017 Jan 1;112:91-104. doi: 10.1016/j.ymeth.2016.09.007. Epub 2016 Sep 15. Methods. 2017. PMID: 27642004 Free PMC article. Review.
Cited by
-
flowDensity: reproducing manual gating of flow cytometry data by automated density-based cell population identification.Bioinformatics. 2015 Feb 15;31(4):606-7. doi: 10.1093/bioinformatics/btu677. Epub 2014 Oct 16. Bioinformatics. 2015. PMID: 25378466 Free PMC article.
-
Automated identification of maximal differential cell populations in flow cytometry data.Cytometry A. 2022 Feb;101(2):177-184. doi: 10.1002/cyto.a.24503. Epub 2021 Oct 22. Cytometry A. 2022. PMID: 34559446 Free PMC article.
-
Competitive SWIFT cluster templates enhance detection of aging changes.Cytometry A. 2016 Jan;89(1):59-70. doi: 10.1002/cyto.a.22740. Epub 2015 Oct 6. Cytometry A. 2016. PMID: 26441030 Free PMC article.
-
Critical assessment of automated flow cytometry data analysis techniques.Nat Methods. 2013 Mar;10(3):228-38. doi: 10.1038/nmeth.2365. Epub 2013 Feb 10. Nat Methods. 2013. PMID: 23396282 Free PMC article.
-
The race to understand immunopathology in COVID-19: Perspectives on the impact of quantitative approaches to understand within-host interactions.Immunoinformatics (Amst). 2023 Mar;9:100021. doi: 10.1016/j.immuno.2023.100021. Epub 2023 Jan 8. Immunoinformatics (Amst). 2023. PMID: 36643886 Free PMC article. Review.
References
-
- Hawley TS, Hawley RG. Flow Cytometry Protocols, Methods in Molecular Biology. 2. Humana Press; 2005.
Publication types
MeSH terms
Grants and funding
LinkOut - more resources
Full Text Sources
Other Literature Sources
Molecular Biology Databases
Miscellaneous