Sleep-spindle detection: crowdsourcing and evaluating performance of experts, non-experts and automated methods
- PMID: 24562424
- PMCID: PMC3972193
- DOI: 10.1038/nmeth.2855
Sleep-spindle detection: crowdsourcing and evaluating performance of experts, non-experts and automated methods
Abstract
Sleep spindles are discrete, intermittent patterns of brain activity observed in human electroencephalographic data. Increasingly, these oscillations are of biological and clinical interest because of their role in development, learning and neurological disorders. We used an Internet interface to crowdsource spindle identification by human experts and non-experts, and we compared their performance with that of automated detection algorithms in data from middle- to older-aged subjects from the general population. We also refined methods for forming group consensus and evaluating the performance of event detectors in physiological data such as electroencephalographic recordings from polysomnography. Compared to the expert group consensus gold standard, the highest performance was by individual experts and the non-expert group consensus, followed by automated spindle detectors. This analysis showed that crowdsourcing the scoring of sleep data is an efficient method to collect large data sets, even for difficult tasks such as spindle identification. Further refinements to spindle detection algorithms are needed for middle- to older-aged subjects.
Conflict of interest statement
All authors report no conflicts of interest.
Figures
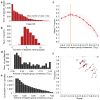
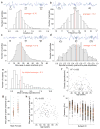
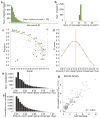
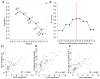
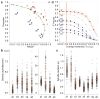
Similar articles
-
On the objectivity, reliability, and validity of deep learning enabled bioimage analyses.Elife. 2020 Oct 19;9:e59780. doi: 10.7554/eLife.59780. Elife. 2020. PMID: 33074102 Free PMC article.
-
Defining the optimum strategy for identifying adults and children with coeliac disease: systematic review and economic modelling.Health Technol Assess. 2022 Oct;26(44):1-310. doi: 10.3310/ZUCE8371. Health Technol Assess. 2022. PMID: 36321689 Free PMC article.
-
Brain tumor segmentation and detection in MRI using convolutional neural networks and VGG16.Cancer Biomark. 2025 Mar;42(3):18758592241311184. doi: 10.1177/18758592241311184. Epub 2025 Apr 4. Cancer Biomark. 2025. PMID: 40183298
-
The 2023 Latin America report of the Lancet Countdown on health and climate change: the imperative for health-centred climate-resilient development.Lancet Reg Health Am. 2024 Apr 23;33:100746. doi: 10.1016/j.lana.2024.100746. eCollection 2024 May. Lancet Reg Health Am. 2024. PMID: 38800647 Free PMC article. Review.
-
Exploring conceptual and theoretical frameworks for nurse practitioner education: a scoping review protocol.JBI Database System Rev Implement Rep. 2015 Oct;13(10):146-55. doi: 10.11124/jbisrir-2015-2150. JBI Database System Rev Implement Rep. 2015. PMID: 26571290
Cited by
-
A comparison of two sleep spindle detection methods based on all night averages: individually adjusted vs. fixed frequencies.Front Hum Neurosci. 2015 Feb 17;9:52. doi: 10.3389/fnhum.2015.00052. eCollection 2015. Front Hum Neurosci. 2015. PMID: 25741264 Free PMC article.
-
Test Expectation Enhances Memory Consolidation across Both Sleep and Wake.PLoS One. 2016 Oct 19;11(10):e0165141. doi: 10.1371/journal.pone.0165141. eCollection 2016. PLoS One. 2016. PMID: 27760193 Free PMC article.
-
Features of the EEG Pattern of Sleep Spindles and Its Diagnostic Significance in Ontogeny.Bull Exp Biol Med. 2022 Aug;173(4):399-408. doi: 10.1007/s10517-022-05557-5. Epub 2022 Sep 5. Bull Exp Biol Med. 2022. PMID: 36058972 Review.
-
Sleep-dependent prospective memory consolidation is impaired with aging.Sleep. 2021 Sep 13;44(9):zsab069. doi: 10.1093/sleep/zsab069. Sleep. 2021. PMID: 33755184 Free PMC article. Clinical Trial.
-
Sleep spindle alterations in patients with Parkinson's disease.Front Hum Neurosci. 2015 May 1;9:233. doi: 10.3389/fnhum.2015.00233. eCollection 2015. Front Hum Neurosci. 2015. PMID: 25983685 Free PMC article.
References
-
- Iber C, Ancoli-Israel S, Chesson A, Quan SF. AASM Manual for the Scoring of Sleep and Associated Events. 2007.
-
- Silverstein LD, Levy CM. The stability of the sigma sleep spindle. Electroencephalogr Clin Neurophysiol. 1976;40:666–670. - PubMed
-
- Tan X, Campbell IG, Feinberg I. Internight reliability and benchmark values for computer analyses of non-rapid eye movement (NREM) and REM EEG in normal young adult and elderly subjects. Clin Neurophysiol. 2001;112:1540–1552. - PubMed
-
- Werth E, Achermann P, Dijk DJ, Borbély AA. Spindle frequency activity in the sleep EEG: individual differences and topographic distribution. Electroencephalogr Clin Neurophysiol. 1997;103:535–542. - PubMed
-
- De Gennaro L, Ferrara M, Vecchio F, Curcio G, Bertini M. An electroencephalographic fingerprint of human sleep. Neuroimage. 2005;26:114–122. - PubMed
Publication types
MeSH terms
Grants and funding
LinkOut - more resources
Full Text Sources
Other Literature Sources