Controllability of structural brain networks
- PMID: 26423222
- PMCID: PMC4600713
- DOI: 10.1038/ncomms9414
Controllability of structural brain networks
Abstract
Cognitive function is driven by dynamic interactions between large-scale neural circuits or networks, enabling behaviour. However, fundamental principles constraining these dynamic network processes have remained elusive. Here we use tools from control and network theories to offer a mechanistic explanation for how the brain moves between cognitive states drawn from the network organization of white matter microstructure. Our results suggest that densely connected areas, particularly in the default mode system, facilitate the movement of the brain to many easily reachable states. Weakly connected areas, particularly in cognitive control systems, facilitate the movement of the brain to difficult-to-reach states. Areas located on the boundary between network communities, particularly in attentional control systems, facilitate the integration or segregation of diverse cognitive systems. Our results suggest that structural network differences between cognitive circuits dictate their distinct roles in controlling trajectories of brain network function.
Figures
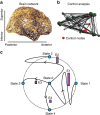
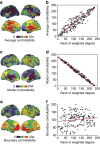
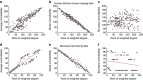
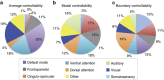
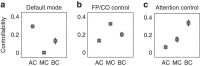
Similar articles
-
Heritability and Cognitive Relevance of Structural Brain Controllability.Cereb Cortex. 2020 May 14;30(5):3044-3054. doi: 10.1093/cercor/bhz293. Cereb Cortex. 2020. PMID: 31838501 Free PMC article.
-
Organization, development and function of complex brain networks.Trends Cogn Sci. 2004 Sep;8(9):418-25. doi: 10.1016/j.tics.2004.07.008. Trends Cogn Sci. 2004. PMID: 15350243 Review.
-
Structural Differences Between Healthy Subjects and Patients With Schizophrenia or Schizoaffective Disorder: A Graph and Control Theoretical Perspective.Front Psychiatry. 2021 Jun 28;12:669783. doi: 10.3389/fpsyt.2021.669783. eCollection 2021. Front Psychiatry. 2021. PMID: 34262489 Free PMC article.
-
Human cognition involves the dynamic integration of neural activity and neuromodulatory systems.Nat Neurosci. 2019 Feb;22(2):289-296. doi: 10.1038/s41593-018-0312-0. Epub 2019 Jan 21. Nat Neurosci. 2019. PMID: 30664771
-
Brain Networks and Cognitive Architectures.Neuron. 2015 Oct 7;88(1):207-19. doi: 10.1016/j.neuron.2015.09.027. Neuron. 2015. PMID: 26447582 Free PMC article. Review.
Cited by
-
Structure-function models of temporal, spatial, and spectral characteristics of non-invasive whole brain functional imaging.Front Neurosci. 2022 Aug 30;16:959557. doi: 10.3389/fnins.2022.959557. eCollection 2022. Front Neurosci. 2022. PMID: 36110093 Free PMC article. Review.
-
Effect of methylphenidate on functional controllability: a preliminary study in medication-naïve children with ADHD.Transl Psychiatry. 2022 Dec 17;12(1):518. doi: 10.1038/s41398-022-02283-4. Transl Psychiatry. 2022. PMID: 36528602 Free PMC article. Clinical Trial.
-
Neuronal Degeneration Impairs Rhythms Between Connected Microcircuits.Front Comput Neurosci. 2020 Mar 3;14:18. doi: 10.3389/fncom.2020.00018. eCollection 2020. Front Comput Neurosci. 2020. PMID: 32194390 Free PMC article.
-
Role of Graph Architecture in Controlling Dynamical Networks with Applications to Neural Systems.Nat Phys. 2018;14:91-98. doi: 10.1038/nphys4268. Epub 2017 Sep 25. Nat Phys. 2018. PMID: 29422941 Free PMC article.
-
Local connectome phenotypes predict social, health, and cognitive factors.Netw Neurosci. 2018 Mar 1;2(1):86-105. doi: 10.1162/NETN_a_00031. eCollection 2018 Spring. Netw Neurosci. 2018. PMID: 29911679 Free PMC article.
References
-
- Botvinick M. M. & Cohen J. D. The computational and neural basis of cognitive control: charted territory and new frontiers. Cogn. Sci. 38, 1249–1285 (2014). - PubMed
-
- Corbetta M. & Shulman G. L. Control of goal-directed and stimulus-driven attention in the brain. Nat. Rev. Neurosci. 3, 201–215 (2002). - PubMed
-
- Cocchi L., Zalesky A., Fornito A. & Mattingley J. B. Dynamic cooperation and competition between brain systems during cognitive control. Trends Cogn. Sci. 17, 493–501 (2013). - PubMed
-
- Bullmore E. & Sporns O. Complex brain networks: graph theoretical analysis of structural and functional systems. Nat. Rev. Neurosci. 10, 186–198 (2009). - PubMed
Publication types
MeSH terms
Grants and funding
LinkOut - more resources
Full Text Sources
Other Literature Sources