Space of preattentive shape features
- PMID: 32315405
- PMCID: PMC7405702
- DOI: 10.1167/jov.20.4.10
Space of preattentive shape features
Abstract
Four decades of studies in visual attention and visual working memory used visual features such as colors, orientations, and shapes. The layout of their featural space is clearly established for most features (e.g., CIE-Lab for colors) but not shapes. Here, I attempted to reveal the basic dimensions of preattentive shape features by studying how shapes can be positioned relative to one another in a way that matches their perceived similarities. Specifically, 14 shapes were optimized as n-dimensional vectors to achieve the highest linear correlation (r) between the log-distances between C (14, 2) = 91 pairs of shapes and the discriminabilities (d') of these 91 pairs in a texture segregation task. These d' values were measured on a large sample (N = 200) and achieved high reliability (Cronbach's α = 0.982). A vast majority of variances in the results (r = 0.974) can be explained by a three-dimensional SCI shape space: segmentability, compactness, and spikiness.
Figures
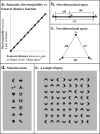
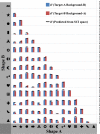
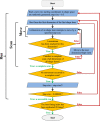
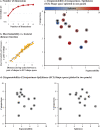

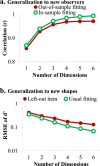
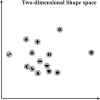
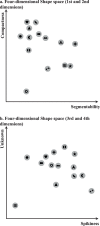
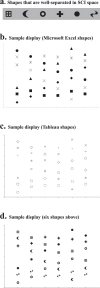
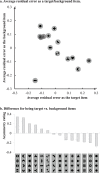


Similar articles
-
Visual features for perception, attention, and working memory: Toward a three-factor framework.Cognition. 2015 Dec;145:43-52. doi: 10.1016/j.cognition.2015.08.007. Epub 2015 Aug 21. Cognition. 2015. PMID: 26299507
-
Different features are stored independently in visual working memory but mediated by object-based representations.Acta Psychol (Amst). 2019 Jun;197:52-63. doi: 10.1016/j.actpsy.2019.05.003. Epub 2019 May 14. Acta Psychol (Amst). 2019. PMID: 31100548
-
What does visual suffix interference tell us about spatial location in working memory?Mem Cognit. 2015 Jan;43(1):133-42. doi: 10.3758/s13421-014-0448-4. Mem Cognit. 2015. PMID: 25030081
-
The precision of visual memory for a complex contour shape measured by a freehand drawing task.Vision Res. 2013 Mar 7;79:17-26. doi: 10.1016/j.visres.2012.12.002. Epub 2013 Jan 4. Vision Res. 2013. PMID: 23296198
-
Association between cue lead time and template-for-rejection effect.Atten Percept Psychophys. 2019 Aug;81(6):1880-1889. doi: 10.3758/s13414-019-01761-0. Atten Percept Psychophys. 2019. PMID: 31114955 Review.
Cited by
-
High-level aftereffects reveal the role of statistical features in visual shape encoding.Curr Biol. 2024 Mar 11;34(5):1098-1106.e5. doi: 10.1016/j.cub.2023.12.039. Epub 2024 Jan 12. Curr Biol. 2024. PMID: 38218184 Free PMC article.
-
Evidence for Independent Processing of Shape by Vision and Touch.eNeuro. 2022 Jun 17;9(3):ENEURO.0502-21.2022. doi: 10.1523/ENEURO.0502-21.2022. Print 2022 May-Jun. eNeuro. 2022. PMID: 35998295 Free PMC article.
-
An image-computable model of human visual shape similarity.PLoS Comput Biol. 2021 Jun 1;17(6):e1008981. doi: 10.1371/journal.pcbi.1008981. eCollection 2021 Jun. PLoS Comput Biol. 2021. PMID: 34061825 Free PMC article.
-
Visual object categorization in infancy.Proc Natl Acad Sci U S A. 2022 Feb 22;119(8):e2105866119. doi: 10.1073/pnas.2105866119. Proc Natl Acad Sci U S A. 2022. PMID: 35169072 Free PMC article.
-
Cue predictiveness and uncertainty determine cue representation during visual statistical learning.Learn Mem. 2023 Nov 3;30(11):282-295. doi: 10.1101/lm.053777.123. Print 2023 Nov. Learn Mem. 2023. PMID: 37923354 Free PMC article.
References
-
- Bae G. Y., & Flombaum J. I. (2013). Two items remembered as precisely as one how integral features can improve visual working memory. Psychological Science, 24, 2038–2047. - PubMed
-
- Beck J. (1982). Texture segregation. In Beck J. (Ed.), Organization and representation in perception (pp. 285–318). Hillsdale, NJ: Erlbaum.
Publication types
MeSH terms
LinkOut - more resources
Full Text Sources
Miscellaneous