Embedding-based Silhouette community detection
- PMID: 33191975
- PMCID: PMC7652809
- DOI: 10.1007/s10994-020-05882-8
Embedding-based Silhouette community detection
Abstract
Mining complex data in the form of networks is of increasing interest in many scientific disciplines. Network communities correspond to densely connected subnetworks, and often represent key functional parts of real-world systems. This paper proposes the embedding-based Silhouette community detection (SCD), an approach for detecting communities, based on clustering of network node embeddings, i.e. real valued representations of nodes derived from their neighborhoods. We investigate the performance of the proposed SCD approach on 234 synthetic networks, as well as on a real-life social network. Even though SCD is not based on any form of modularity optimization, it performs comparably or better than state-of-the-art community detection algorithms, such as the InfoMap and Louvain. Further, we demonstrate that SCD's outputs can be used along with domain ontologies in semantic subgroup discovery, yielding human-understandable explanations of communities detected in a real-life protein interaction network. Being embedding-based, SCD is widely applicable and can be tested out-of-the-box as part of many existing network learning and exploration pipelines.
Keywords: Community detection; Network analysis; Node embedding; Unsupervised learning.
© The Author(s) 2020.
Figures
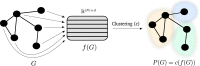
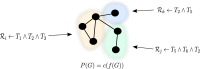
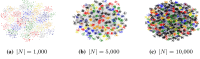
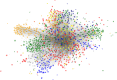
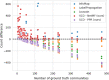
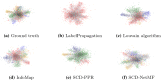
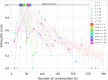
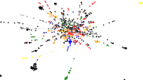
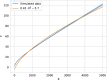
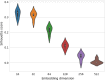
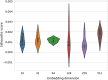
Similar articles
-
Community detection in networks using graph embeddings.Phys Rev E. 2021 Feb;103(2-1):022316. doi: 10.1103/PhysRevE.103.022316. Phys Rev E. 2021. PMID: 33736102
-
Deep Network Embedding for Graph Representation Learning in Signed Networks.IEEE Trans Cybern. 2020 Apr;50(4):1556-1568. doi: 10.1109/TCYB.2018.2871503. Epub 2018 Oct 9. IEEE Trans Cybern. 2020. PMID: 30307885
-
TigeCMN: On exploration of temporal interaction graph embedding via Coupled Memory Neural Networks.Neural Netw. 2021 Aug;140:13-26. doi: 10.1016/j.neunet.2021.02.016. Epub 2021 Mar 4. Neural Netw. 2021. PMID: 33743320
-
Deep Representation Learning for Social Network Analysis.Front Big Data. 2019 Apr 3;2:2. doi: 10.3389/fdata.2019.00002. eCollection 2019. Front Big Data. 2019. PMID: 33693325 Free PMC article. Review.
-
Connecting the dots: The boons and banes of network modeling.Patterns (N Y). 2021 Dec 10;2(12):100374. doi: 10.1016/j.patter.2021.100374. eCollection 2021 Dec 10. Patterns (N Y). 2021. PMID: 34950902 Free PMC article. Review.
Cited by
-
PubMed-Scale Chemical Concept Embeddings Reconstruct Physical Protein Interaction Networks.Front Res Metr Anal. 2021 Apr 13;6:644614. doi: 10.3389/frma.2021.644614. eCollection 2021. Front Res Metr Anal. 2021. PMID: 33928210 Free PMC article.
-
Change in Threads on Twitter Regarding Influenza, Vaccines, and Vaccination During the COVID-19 Pandemic: Artificial Intelligence-Based Infodemiology Study.JMIR Infodemiology. 2021 Oct 14;1(1):e31983. doi: 10.2196/31983. eCollection 2021 Jan-Dec. JMIR Infodemiology. 2021. PMID: 34693212 Free PMC article.
-
Overlapping community detection in weighted networks via hierarchical clustering.PLoS One. 2024 Oct 28;19(10):e0312596. doi: 10.1371/journal.pone.0312596. eCollection 2024. PLoS One. 2024. PMID: 39466771 Free PMC article.
References
-
- Adhikari PR, Vavpetič A, Kralj J, Lavrač N, Hollmén J. Explaining mixture models through semantic pattern mining and banded matrix visualization. Machine Learning. 2016;105(1):3–39.
-
- Aranganayagi, S., & Thangavel, K. (2007). Clustering categorical data using silhouette coefficient as a relocating measure. In International conference on computational intelligence and multimedia applications (ICCIMA 2007) (vol. 2, pp. 13–17). IEEE.
-
- Arthur, D., & Vassilvitskii, S. (2007). k-means++: The advantages of careful seeding. In Proceedings of the eighteenth annual ACM-SIAM symposium on Discrete algorithms (pp. 1027–1035). Society for Industrial and Applied Mathematics.
-
- Bachem, O., Lucic, M., Hassani, H., & Krause, A. (2016). Fast and provably good seedings for k-means. In Advances in neural information processing systems 29 (pp. 55–63). Curran Associates Inc.
LinkOut - more resources
Full Text Sources