Genetic diversity fuels gene discovery for tobacco and alcohol use
- PMID: 36477530
- PMCID: PMC9771818
- DOI: 10.1038/s41586-022-05477-4
Genetic diversity fuels gene discovery for tobacco and alcohol use
Abstract
Tobacco and alcohol use are heritable behaviours associated with 15% and 5.3% of worldwide deaths, respectively, due largely to broad increased risk for disease and injury1-4. These substances are used across the globe, yet genome-wide association studies have focused largely on individuals of European ancestries5. Here we leveraged global genetic diversity across 3.4 million individuals from four major clines of global ancestry (approximately 21% non-European) to power the discovery and fine-mapping of genomic loci associated with tobacco and alcohol use, to inform function of these loci via ancestry-aware transcriptome-wide association studies, and to evaluate the genetic architecture and predictive power of polygenic risk within and across populations. We found that increases in sample size and genetic diversity improved locus identification and fine-mapping resolution, and that a large majority of the 3,823 associated variants (from 2,143 loci) showed consistent effect sizes across ancestry dimensions. However, polygenic risk scores developed in one ancestry performed poorly in others, highlighting the continued need to increase sample sizes of diverse ancestries to realize any potential benefit of polygenic prediction.
© 2022. The Author(s).
Conflict of interest statement
The spouse of N.L. Saccone is listed as an inventor on issued U.S. patent 8080371 ‘Markers of addiction’, covering the use of certain single-nucleotide polymorphisms in determining the diagnosis, prognosis and treatment of addiction. M.H.C. has received grant funding from GSK and Bayer, and speaking or consulting fees from AstraZeneca, Illumina and Genentech. R.T.-S. is a former employee and current shareholder of GSK and is currently a non-executive member of the ENA Respiratory board of directors. She reports personal fees from Teva, Immunomet, Vocalis Health and ENA Respiratory (until January 2021). D.A.S. is the founder and chief scientific officer of Eleven P15, a company focused on the early diagnosis of treatment of pulmonary fibrosis. J.B.N. and E.J. are employed by Regeneron Pharmaceuticals, Inc. The spouse of C.J.W. is employed by Regeneron Pharmaceuticals, Inc. L.J.B. is listed as an inventor on Issued U.S. Patent 8080371 ‘Markers for addiction’, covering the use of certain single-nucleotide polymorphisms in determining the diagnosis, prognosis and treatment of addiction. The 23andMe Research Team, including J.S. and S.S.S., are employees of 23andMe, Inc., and hold stock and/or stock options in 23andMe. T.E.T., D.F.G., H.S., G.B. and K. Stefansson are employees of deCODE genetics/AMGEN. M. Moll received grant support from Bayer. A.W.B. is listed as a co-inventor on a U.S. patent application ‘Biosignature discovery for substance use disorder using statistical learning’ assigned to BioRealm, LLC, and serves as a scientific advisor and consultant to BioRealm, LLC. All other authors declare no competing interests.
Figures
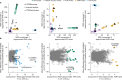
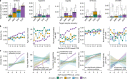
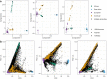
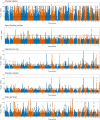
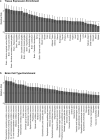
Similar articles
-
Exploring the role of low-frequency and rare exonic variants in alcohol and tobacco use.Drug Alcohol Depend. 2018 Jul 1;188:94-101. doi: 10.1016/j.drugalcdep.2018.03.026. Epub 2018 Apr 25. Drug Alcohol Depend. 2018. PMID: 29758381
-
Ancestry-specific associations identified in genome-wide combined-phenotype study of red blood cell traits emphasize benefits of diversity in genomics.BMC Genomics. 2020 Mar 14;21(1):228. doi: 10.1186/s12864-020-6626-9. BMC Genomics. 2020. PMID: 32171239 Free PMC article.
-
Clinical use of current polygenic risk scores may exacerbate health disparities.Nat Genet. 2019 Apr;51(4):584-591. doi: 10.1038/s41588-019-0379-x. Epub 2019 Mar 29. Nat Genet. 2019. PMID: 30926966 Free PMC article. Review.
-
Cross-ancestry genome-wide association studies identified heterogeneous loci associated with differences of allele frequency and regulome tagging between participants of European descent and other ancestry groups from the UK Biobank.Hum Mol Genet. 2021 Jul 9;30(15):1457-1467. doi: 10.1093/hmg/ddab114. Hum Mol Genet. 2021. PMID: 33890984 Free PMC article.
-
Research review: Polygenic methods and their application to psychiatric traits.J Child Psychol Psychiatry. 2014 Oct;55(10):1068-87. doi: 10.1111/jcpp.12295. Epub 2014 Aug 1. J Child Psychol Psychiatry. 2014. PMID: 25132410 Review.
Cited by
-
Genetic correlation and causal associations between circulating C-reactive protein levels and lung cancer risk.Cancer Causes Control. 2024 Jun;35(6):897-906. doi: 10.1007/s10552-024-01855-7. Epub 2024 Feb 8. Cancer Causes Control. 2024. PMID: 38332239
-
Sex differences in risk factor relationships with subarachnoid haemorrhage and intracranial aneurysms: A Mendelian randomization study.Eur Stroke J. 2024 Jul 30;10(1):216-224. doi: 10.1177/23969873241265224. eCollection 2025 Mar. Eur Stroke J. 2024. PMID: 39081091 Free PMC article.
-
The causality between smoking and intervertebral disc degeneration mediated by IL-1β secreted by macrophage: A Mendelian randomization study.Heliyon. 2024 Aug 28;10(17):e37044. doi: 10.1016/j.heliyon.2024.e37044. eCollection 2024 Sep 15. Heliyon. 2024. PMID: 39286222 Free PMC article.
-
A comprehensive analysis of the health effects associated with smoking in the largest population using UK Biobank genotypic and phenotypic data.Heliyon. 2024 Aug 2;10(16):e35649. doi: 10.1016/j.heliyon.2024.e35649. eCollection 2024 Aug 30. Heliyon. 2024. PMID: 39220930 Free PMC article.
-
Smoking, alcohol consumption, and frailty: A Mendelian randomization study.Front Genet. 2023 Feb 3;14:1092410. doi: 10.3389/fgene.2023.1092410. eCollection 2023. Front Genet. 2023. PMID: 36816044 Free PMC article.
References
-
- World Health Organization. Tobacco. WHOhttps://www.who.int/news-room/fact-sheets/detail/tobacco (2022).
-
- World Health Organization. Alcohol. WHOhttps://www.who.int/news-room/fact-sheets/detail/alcohol (2022).
-
- World Health Organization. The top 10 causes of death. WHOhttps://www.who.int/news-room/fact-sheets/detail/the-top-10-causes-of-death (2020).
Publication types
MeSH terms
Grants and funding
- R01 DK093757/DK/NIDDK NIH HHS/United States
- R21 DA040177/DA/NIDA NIH HHS/United States
- R44 AA027675/AA/NIAAA NIH HHS/United States
- R56 HG011035/HG/NHGRI NIH HHS/United States
- R01 HG008983/HG/NHGRI NIH HHS/United States
- K24 HL148521/HL/NHLBI NIH HHS/United States
- MC_U137686851/MRC_/Medical Research Council/United Kingdom
- R01 NS114045/NS/NINDS NIH HHS/United States
- P30 CA014236/CA/NCI NIH HHS/United States
- R03 HL154284/HL/NHLBI NIH HHS/United States
- MC_PC_14135/MRC_/Medical Research Council/United Kingdom
- T32 DA050560/DA/NIDA NIH HHS/United States
- R01 HG011035/HG/NHGRI NIH HHS/United States
- MC_QA137853/MRC_/Medical Research Council/United Kingdom
- MC_PC_17228/MRC_/Medical Research Council/United Kingdom
- R37 AA009367/AA/NIAAA NIH HHS/United States
- U01 DA041120/DA/NIDA NIH HHS/United States
- G0902144/MRC_/Medical Research Council/United Kingdom
- R56 HG012358/HG/NHGRI NIH HHS/United States
- 212946/Z/18/Z/WT_/Wellcome Trust/United Kingdom
- R03 OD032630/OD/NIH HHS/United States
- R01 EB015611/EB/NIBIB NIH HHS/United States
- 29186/CRUK_/Cancer Research UK/United Kingdom
- MC_UU_12026/2/MRC_/Medical Research Council/United Kingdom
- R01 GM126479/GM/NIGMS NIH HHS/United States
- R01 HL077612/HL/NHLBI NIH HHS/United States
- R01 HL153805/HL/NHLBI NIH HHS/United States
- R01 DK072193/DK/NIDDK NIH HHS/United States
- R01 HL093081/HL/NHLBI NIH HHS/United States
- R01 HL105756/HL/NHLBI NIH HHS/United States
- MC_UU_00017/1/MRC_/Medical Research Council/United Kingdom
- R01 DA042755/DA/NIDA NIH HHS/United States
- P30 ES030285/ES/NIEHS NIH HHS/United States
- MR/S003762/1/MRC_/Medical Research Council/United Kingdom
- MC_PC_13049/MRC_/Medical Research Council/United Kingdom
- P01 HL132825/HL/NHLBI NIH HHS/United States
- U54 GM115428/GM/NIGMS NIH HHS/United States
- P30 CA071789/CA/NCI NIH HHS/United States
- R01 HL149683/HL/NHLBI NIH HHS/United States
- R01 DA044283/DA/NIDA NIH HHS/United States